Members of iHEALTH participated in the Annual Conference of the Association for Computational Linguistics (ACL)
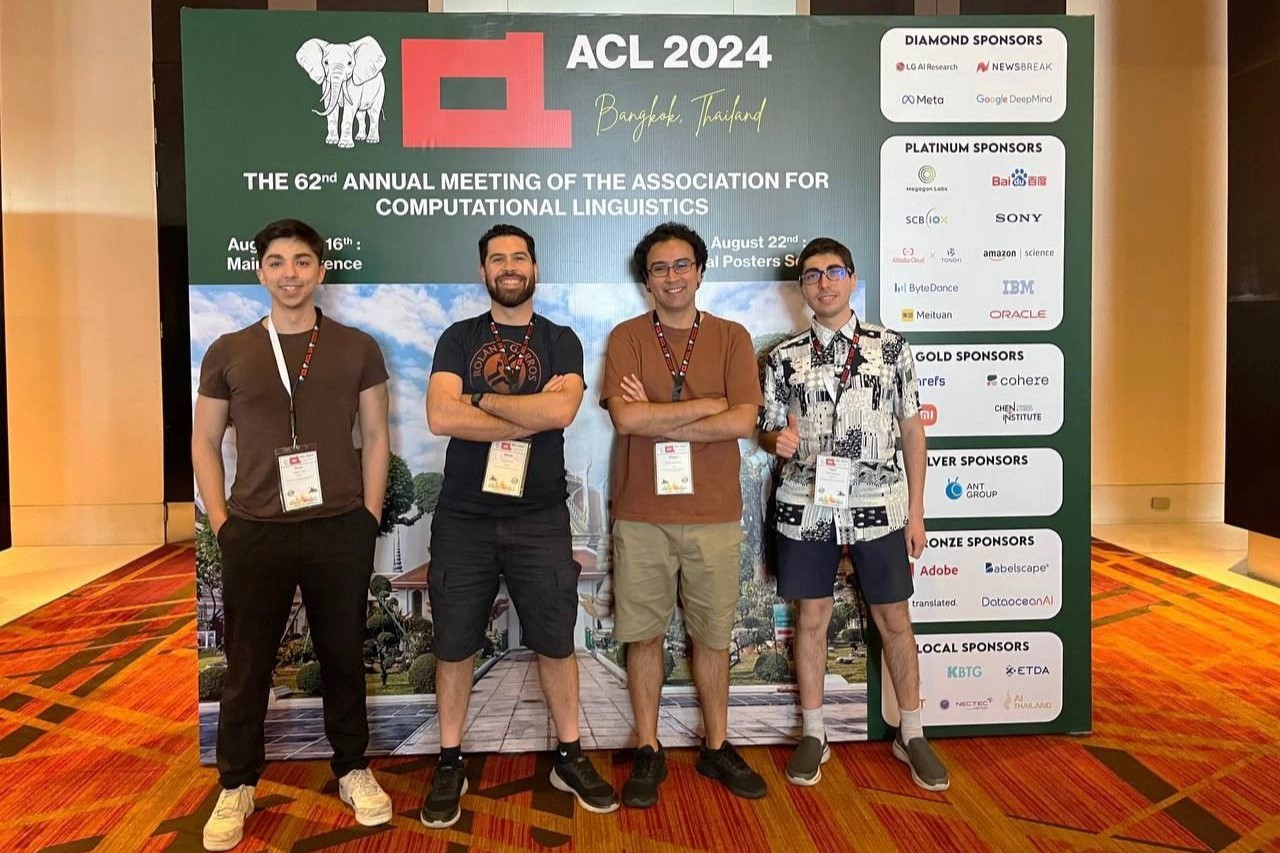
Dr. Denis Parra, principal investigator at iHEALTH; Diego Campanini, a young researcher at the institute; and Oscar Loch and Pablo Messina, Ph.D. students at Pontificia Universidad Católica de Chile, participated in the event held in Bangkok, Thailand.
The 62nd Annual Meeting of the Association for Computational Linguistics (ACL) this year aimed to foster discussions on open science and reproducible research in natural language processing (NLP), as well as support the open-source software movement.
Members of the Millennium Institute iHEALTH presented at the annual conference to showcase their recent work on the application of NLP for radiology report generation.
Pablo Messina was the first to present with the work in progress, “Extracting and Encoding: Leveraging Large Language Models and Medical Knowledge to Enhance Radiological Text Representation.”
In an innovative approach to improve representation learning in medicine, the study developed a two-stage framework that extracts high-quality factual statements from free-text radiology reports. This framework includes a "Fact Extractor" that uses large language models (LLMs) to identify domain-specific data and a "Fact Encoder" based on a BERT model, which enhances text representation using these data. Additionally, a new embedding-based metric (CXRFEScore) was introduced, proving to be more robust and effective than existing metrics, improving the evaluation of text generation systems in radiology and outperforming current methods in key tasks.
Diego Campanini presented the study“In-context Learning and Finetuning of a Large Multimodal Model for Radiology Report Generation", focusing on large-scale radiology report generation, highlighting the use of the LLaVA model, which combines a vision encoder, a vision-language connector, and a large language model. By enriching the input with text generated by a simpler model, they improved performance on 4 out of 5 key metrics. The study also detailed architectural settings, fine-tuning strategies, and data configurations to optimize results.
On the other hand, Oscar Loch and Pablo Messina presented their results in the poster “Template Based Report Generation” where they showcased another template-based strategy for large-scale radiology report generation, using predictions from multi-label image classifiers as input. This technique achieved an F1-RadGraph score of 19.42, although discrepancies were observed between classification metrics and the F1-CheXbert metric, suggesting potential limitations in label capture by the latter. The approach underscores the need to improve evaluation metrics to more accurately reflect the quality of generated reports.
Regarding the event, Dr. Denis Parra mentioned: "An excellent workshop and poster session, we leave with several lessons, new contacts, and work to do to advance our research in AI + Health."