Seminar May 14TH, 2024
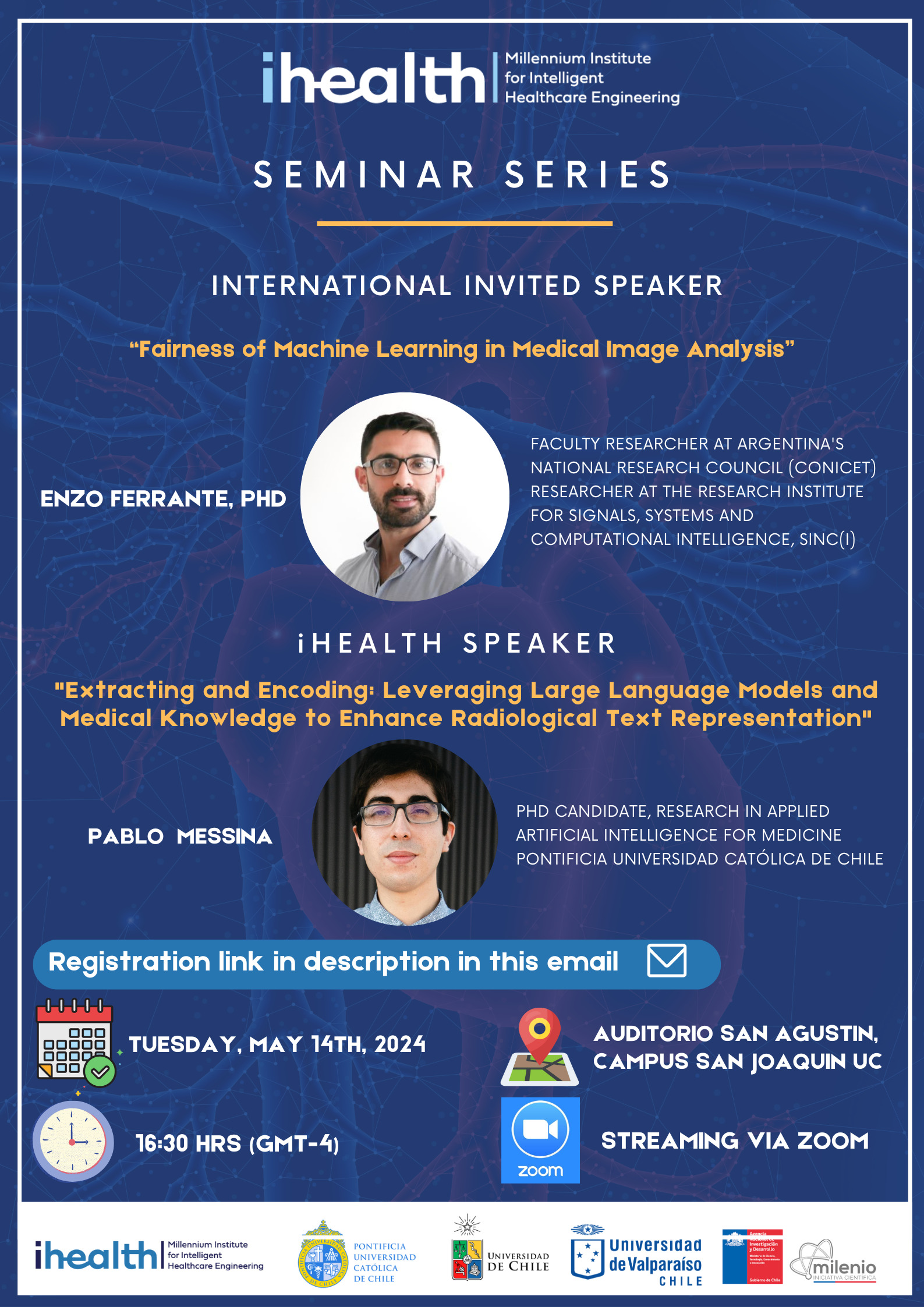
Hours: 16:30 - 18:00
INTERNATIONAL INVITED SPEAKER
Enzo Ferrante, PhD
TITLE: Fairness of machine learning in medical image analysis
ABSTRACT: Medical institutions around the world are adopting machine learning (ML) systems to assist in analyzing health data; at the same time, the research community of fairness in ML has shown that these systems can be biased, resulting in disparate performance for specific subpopulations. In this talk, we will discuss the relationship between bias, ML and health systems, addressing the specific case of gender bias in X-ray classifiers [1] for computer-assisted diagnosis [2].
SHORT BIO: Dr. Enzo Ferrante completed his PhD in Computer Sciences at Université Paris-Saclay (Paris, France) and worked as a postdoctoral researcher at Imperial College London in the UK. He has also been a visiting student at the CVGL Lab at Stanford University (2014), Fulbright Visiting Researcher at the A. Martinos Center for Biomedical Imaging (Massachusetts General Hospital - Harvard Medical School, 2021) in Boston, and DATAIA Invited Professor position at Université Paris-Saclay in France (2023-2024).
He returned to Argentina in 2017, holding a faculty researcher position at Argentina's National Research Council (CONICET). He regularly serves as a member of the program committee of important medical imaging conferences like MICCAI, MIDL, ISBI and IPMI, and he has organized several workshops in this context. He leads the Machine Learning for Biomedical Image Computing group in the Research Institute for Signals, Systems and Computational Intelligence, sinc(i). Dr Ferrante received the Google Award for Inclusion Research (2022), the Young Researcher Award from the National Academy of Sciences of Argentina (2020) and the Mercosur Science & Technology Award (2020) for his scientific contributions to AI for medical imaging and fairness of AI in this context. His research interests span artificial intelligence and biomedical image analysis, currently focusing on fairness, domain adaptation and generalization, calibration and anatomical segmentation of medical image.
iHEALTH SPEAKER
Pablo Messina Gallardo
TITLE: Extracting and Encoding: Leveraging Large Language Models and Medical Knowledge to Enhance Radiological Text Representation
ABSTRACT: Recent advancements in representation learning face challenges in specialized domains like medicine, where acquiring expert annotations for texts and images is burdensome due to the limited availability of medical professionals. To address this, we propose a two-stage framework aimed at extracting factual statements to improve encoder representations for various downstream tasks. First, we introduce a Fact Extractor that leverages the effectiveness of large language models in the medical domain to obtain factual statements from well-curated domain-specific datasets. In the second stage, we present a Fact Encoder (CXRFE) based on a BERT model that we fine-tune with objective functions designed to enhance its representations using the gathered data. Additionally, our framework introduces a novel embedding-based metric (CXRFEScore) for evaluating chest X-ray text generation systems, utilizing the two stages of our framework. Through extensive evaluations, we demonstrate that our fact extractor and encoder outperform multiple state-of-the-art methods across tasks such as sentence ranking, natural language inference, and label extraction from radiology reports. Furthermore, we show that our metric is robust, outperforming existing metrics commonly used in the report generation literature.
SHORT BIO: Pablo Messina is a sixth-year doctoral student at the Pontificia Universidad Católica de Chile. His supervising professors are Denis Parra (iHEALTH, CENIA) and Álvaro Soto (CENIA). His thesis focuses on the problem of automating the generation of radiological reports from chest X-ray images, using neural networks and an approach explainable through questions and answers.