Seminar March 26TH, 2024
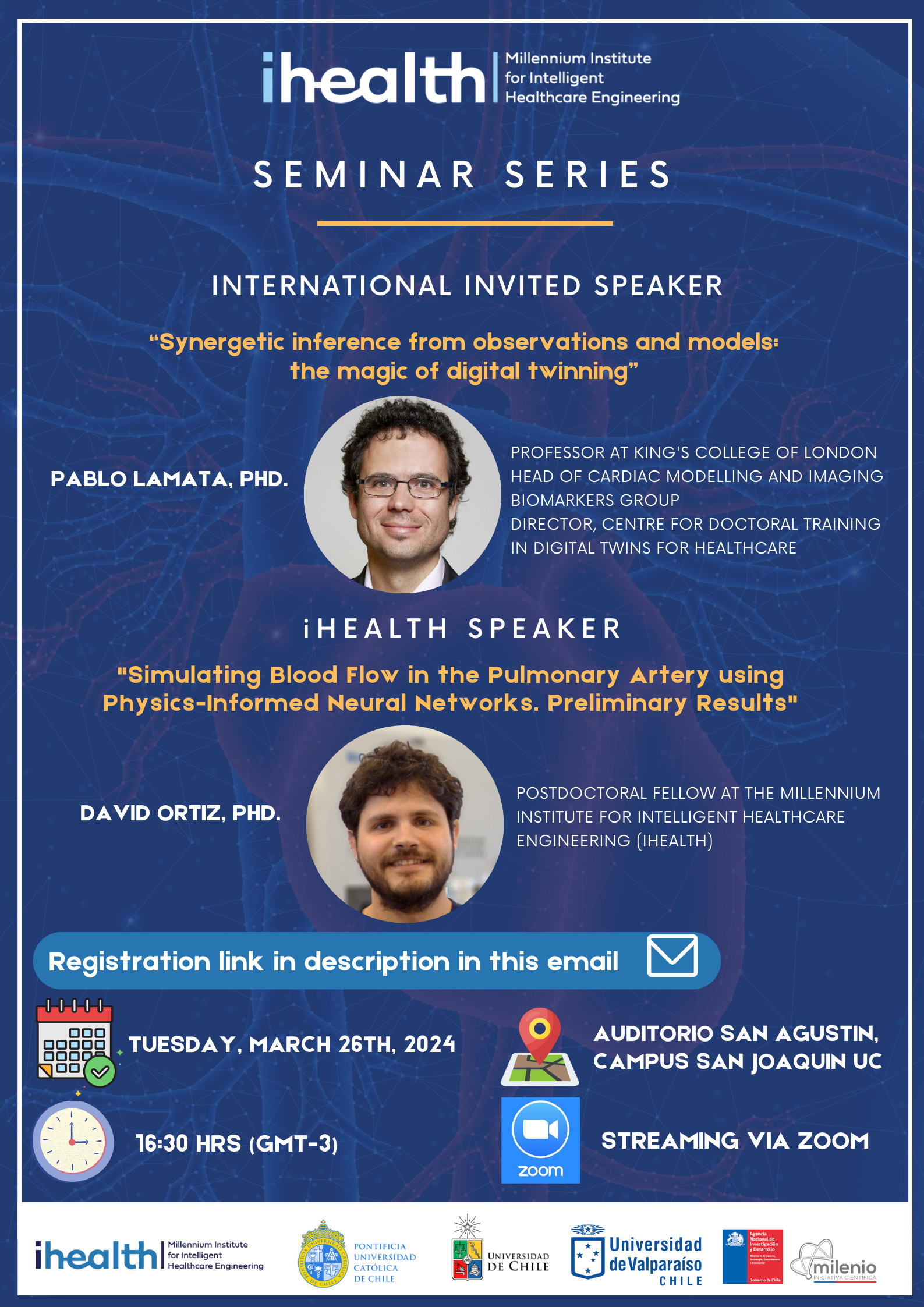
Hours: 16:30 - 18:00
PABLO LAMATA, PHD.
TITLE: “Synergetic inference from observations and models: the magic of digital twinning”.
ABSTRACT: Predictions. Inference of future (health-related) events to then plan how to avoid them. And inference of current hidden diagnosis to then plan how to minimise future risks. That’s the core of medical decision-making, and what a digital twin of a patient can best support - its magic is the ability to combine the strengths of statistical and mechanistic models. This talk will present this concept of the digital twin, illustrate it through old and novel examples already deployed in clinical practice, and review some of the latest research efforts from the team at King’s College London. A discussion of the opportunities and challenges in this exciting area will finally set the scene to a hopefully engaging Q&A session.
BIO: Pablo is a professor in biomedical engineering at King’s College of London. He focuses his research interest on the combination of imaging and computational modelling technologies to improve the management of cardiovascular diseases. His team (http://cmib.website) has developed solutions for the identification of faulty valves, for the detection of growth differences caused by pre-term birth, or for the optimal patient selection for ablation or resynchronization therapies, among others. He is the director of the Centre for Doctoral Training in Digital Twins for Healthcare (https://www.dt4health.org). Pablo has developed his academic career at King’s, Oxford, Madrid and Zaragoza. He has worked at Siemens, and lived an entrepreneurial adventure called Congenita Ltd. He strives to make a real impact in the provision of better healthcare solutions.
DAVID ORTIZ, PHD.
TITLE: “Simulating Blood Flow in the Pulmonary Artery using Physics-Informed Neural Networks. Preliminary Results”.
ABSTRACT: Computational modeling of Pulmonary arterial hypertension (PAH) poses significant challenges due to the complex dynamics of blood flow within the pulmonary artery. In order to study these complex hemodynamics, we propose utilizing Physics-Informed Neural Networks (PINN) along with the Navier-Stokes equations to simulate these intricate flow patterns. Our methodology seeks to leverage the strength of PINN to encode physical laws directly into the neural network and perform data-independent forward simulations, promising a robust framework for simulating physiological flows under pathological conditions. Preliminary results show high accuracy against well-known PDE models. However, when compared directly to benchmarks based on the Navier-Stokes equations, our model reveals limitations, particularly in replicating complex flow phenomena such as vortex shedding. These findings highlight the need for further refinement to model PAH-specific flows accurately. Future work will focus on adjusting the PINN framework to represent better the complex physics of vortex shedding and other flow phenomena, aiming to close the gap identified
through these benchmark comparisons.
BIO: David Ortiz is a postdoctoral fellow at the Millennium Institute for Intelligent Healthcare Engineering (iHEALTH) in Chile. He focuses on applying physics-informed machine-learning techniques to blood flow simulations and inverse problems in the pulmonary artery. With a Ph.D. in engineering sciences from the Pontifical Catholic University of Chile, his research centers on integrating advanced image processing techniques like filtering, segmentation, and registration with biomechanics, finite element methods, and physics-informed machine learning. This integration aims to enhance tools for the early and precise diagnosis of different diseases.